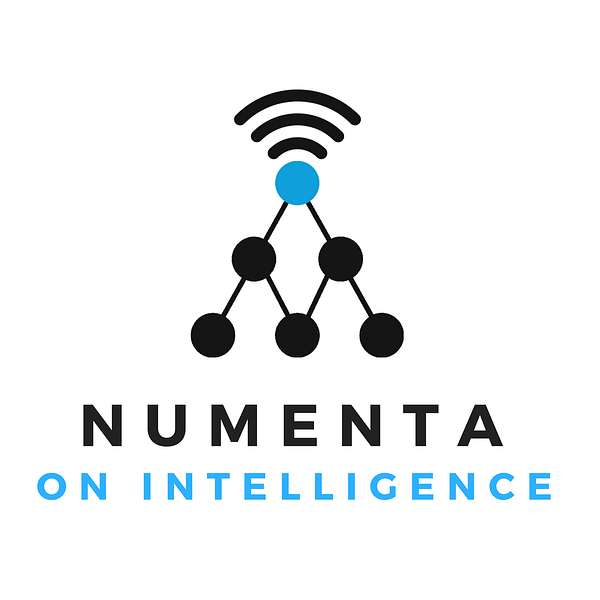
Numenta On Intelligence
Numenta On Intelligence
Episode 8: Interview with a Neuroscientist - Konrad Kording Part 1
Dr. Konrad Kording is a professor at University of Pennsylvania, known for his contributions to the fields of motor control, neural data methods, and computational neuroscience. He runs the Kording Lab, or K-Lab, which focused on computational neuroscience early on and now focuses on causality in data science applications. His lab has made an impact across many fields over time, including Bayesian brains, causal effects in human behavior, and uncertainty in the brain.
In this episode, Matt Taylor interviews Dr. Kording over Skype to discuss illusions of perception, how uncertainty might be represented in the brain, and more. A video of their conversation is available on the HTM School YouTube channel.
When I don't show you your hands, you don't see your hand for a while, it turns out that you become uncertain of where your hand is because your proprioception has this thing that's called"drift." So basically if I rotate your hand here and hold it long enough, you will kind of no longer know exactly where it is.
Matt:Welcome back to Numenta On Intelligence podcast and another Interview with a Neuroscientist. A little clip for my interview with Dr. Konrad Kording, who I'll be talking to today. This is going to be part one of a two-part series, and I'm also making some video available of this conversation that we had via Skype, so you can find that on the HTM School YouTube channel. There are a couple of communication blips during this conversation that hopefully your brain's going to work around and understanding what we are saying. Otherwise, we will have part two for you in February, so I hope you will listen in then. Here's Dr. Konrad Kording. Okay. Yeah, I think it's because I turned the recording on.
Konrad:Okay. Hello. Recording! And it says you are recording the call. Great. Cool. Well, nice talking with you. So, what exactly is the objective? I always try to see what's driving the whole thing.
Matt:So my drive is a couple of things. First, I'm a community manager for Numenta and I'm trying to foster this community of hobbyists, scientists, engineers. It's a very diverse community of people, you know. When you just open something up like this, you get a lot of interesting perspectives. But my main focus is education, and I really am trying to focus young people. With this series of interviews, in general, I'd like to try and engage people to be interested in how intelligence works and how the brain is implementing it and things like that because that's what got me into it, right? So I'm sort of focusing on the new generation of AI experts as they're growing and I really want them to learn how intelligence works, not just the Bayesian models necessarily, you know.
Konrad:Well, of course.
Matt:And I'm focusing on how my company is trying to understand the brain and the discoveries that we think we're making, and the theory that the way we think it works because it's theory. It's theory, and we're not doing experiments, but we're always looking at the experimental data to make sure that the theory still works, you know, in the context of these new experiments and stuff coming up. And the reason I came to you is because you're sort of the type of neuroscientists now that the way you think about the brain really aligns well with the way we think about the brain.
Konrad:That's great to hear. I mean, as you know, I come from a Bayesian background. Right now, I might be a little more connectionist than Bayesian.
Matt:Ebbs and flows.
Konrad:But you can't get the Bayesian out of thinking. For me, I see everything through a Bayesian lens even if I'm not doing Bayesian things anymore.
Matt:That's totally different, and that's great because that's a perspective that I can't present to my audience because I didn't come from that perspective. By trade, I'm a software engineer. I didn't learn any of the maths involved. I just learned how to build things with software, right? So I came out of this from an engineering standpoint, how do I build intelligence? That was my interest, like what if something is intelligent and we can figure out how to reverse engineer it and build it? So that's really the direction we're coming from and we're meeting in the middle. I mean, eventually we're all going to meet in the middle and build something amazing. Like that's the vision, right?
Konrad:You're right. You know, you should have started the interview. It starts to be fun. Like people might find these topics interesting already.
Matt:We may as well throw the sentiment. It's recording.
Konrad:Awesome. Cool.
Matt:So, great to meet you by the way. And I haven't introduced you to the audience or anything but since we're already recording, the interview's probably started. Everyone, this is Dr. Konrad Kording. I don't have a good intro. Let me let you introduce yourself. I find that it's better for people anyway, because I always mess it up.
Konrad:Well, I am not sure if I am good at introducing myself. So I'm a neuroscientist. I'm a failed experimentalist. I started my PhD recording from primary visual cortex of the cat, didn't work all that well, so I ended up thinking of how we can use math and computation to understand the brain. And I looked at the brain from many different perspectives over time. I was Bayesian for a long time and thinking about neural computing my entire life, in a way. I'm interested in deep learning and how the brain might be like deep learning and how it might be different. And beyond neuroscience, I'm interested in what we can meaningfully say about the world using data. I'm bad at introducing myself in that way. I'm just interested in whatever is interesting.
Matt:That's a good way to put it. I mean, I'm the same way. I just haven't taken the PhD track, and a lot of people in our community are the same. We're very passionate about trying to understand how things work. But there's no major– I get asked this a lot in our community– What should I study if I want to understand how intelligence works? And this next sort of era of AI, what should I study? Computational neuroscience or straight up neuroscience or go straight with math and physics? And it's hard to say, really.
Konrad:But I think the answer is yes.
Matt:Yeah, exactly.
Konrad:You should probably study all of them at some level. If you don't understand psychology, it's hard for you to get what intelligence is about. If you don't study math, it's very hard to make what you want to say concrete. If you don't study computation, you can't actually implement it and lots of problems you only see it once you've tried to build it. And similarly, methods from physics, they just help you think about the brain in a broader way, I think.
Matt:It took me a very long time to finally come to a somewhat of a basic understanding of intelligence, and even that is very broad and abstract.
Konrad:Please share it. I'm sometimes very unclear about how the brain works.
Matt:Oh gosh, don't put me on the spot. I'm interviewing you! So let me flip it on you, because you said you used to be a Bayesian that sort of like, maybe you've changed your view a bit. One of the things I found that was a theme in your writing or in the papers that you've produced is that if you structure your experiment in the right way, it's amazing how much data we can get now from experiments and it's just getting more and more. And because it's so messy, sometimes you can structure your experiment that you will find what you're looking for sometimes even though it may not be what you're looking for. Right? Maybe you could talk a bit about that.
Konrad:Yeah. I mean, often times in behavioral experiments, what you're looking for is some kind of effect, say"Is uncertainty relevant when you make decisions?" So I give you a task, where uncertainty's really useful, like you knowing how uncertain you are is really essential. So that's one thing you should do if you're very uncertain and another thing that you should do if you're very certain about the situation, and everything else will be the same. So if you want only uncertainty matters and then yes, you use uncertainty, and we've shown that in in many experiments. But if I give you a situation where the reward matters and nothing else, you'll look for the reward. If I give you a situation, where at some level arousal matters or something, like how much you're engaged in a task, well then that will matter. If I give you a task where say color matters, well then color matters. So at some level of uncertainty, there was like in the early two thousands, like 2005, maybe up to 2010. There were all of a sudden lots of labs that said, well let's see if uncertainty is important, and they all found that uncertainty is important. But if we had, instead, gotten all excited about color, then we might have had brain theories that centered around the idea of color. So in the end, when these fields really started going, the question is really what we didn't know in the end. Like we now know that uncertainty matters, sure. If I asked you how certain are you that you will get up between 6:00 AM and 9:00 AM tomorrow, you'll give me a pretty precise answer to that. If I asked you how certain it is that I'll get up in that interval, which you'll have more uncertainty, but I'm sure you'll be less precise.
Matt:Right.
Konrad:And once I give you some information, you'll be better at that. So my view is very much based on uncertainty as that center piece of the way intelligence works, and you can tell a story where everything you do is about uncertainty. Now you can tell another story, which is everything is about learning. This is a story that you can tell just as well, where you could say, well if you make a mistake, if something goes wrong, you'll change and next time you'll be better. Now that view can equally, in a way, explain a lot of intelligence, including what uncertainty gives us. So uncertainty says along with something that I know I kind of store how certain I am about it, which means that if I'm very uncertain about something, I will rely less on the thing than if I'm very certain about something. Now a learning system will do the same thing because it figures out that in the cases where it was very certain but ignored the things, it did worse than the cases where it very certain and use the things in the uncertain cases, but it was very uncertain about things and very much relied on it. That was a mistake. And so therefore, where are we on that continuum? I'm not sure. It could be that the brain– we're born as people who are there to deal with uncertainty because uncertainty is so important, in which case if you want uncertainties don't enter the plan with which our brain is made. Or alternatively, we could be really good at learning and we would never know the difference.
Matt:Would you say that uncertainty is sort of one dimension of an aspect of our brain or it applies to all and lots of other things that are that are happening there, or?
Konrad:Yeah, I think it is an aspect of reasoning. It's an important aspect of reasoning. Like things that you are more uncertain about are less important in the way. And if you have a bunch of things that you're somewhat uncertain about, each of them you can combine them to become more certain.
Matt:That's problem solving.
Konrad:That's problem solving, exactly. And dealing with uncertainty is an unavoidable aspect of intelligence, of problem solving.
Matt:Right. I visualize this a lot in my head. It's hard, right? When I think about ideas and objects or things, discrete things that we think about, you can apply uncertainty to it. I like to think about those things as multidimensional attractors, like there's some match in your brain and some certain neurons that fire when you think about a specific thing, and the uncertainty about that is sort of how messy and noisy is that attractor, how well defined is that thing in your neurons and the connections between them.
Konrad:That is one way how the brain could deal with uncertainty. It's not the only way how you could deal with uncertainty. Let's say, it's possible that kind of, let's say we have some estimation– is that thing behind you a guitar that I see? Now, let's say if my video was somewhat blocked, it could be that it's a guitar or could also just be that it's like a painting or something behind you. So there's two very different ways how the brain could represent such a thing. It could either be, if I'm uncertain, if let's say the image quality is low, my brain activity is very messy. But alternatively my brain activity could be absolutely not messy at all, where I could say this could be a guitar, exactly this guitar with probability 0.9, or this could be not messy at all. I could say guitar, not guitar. And so at some level, this messiness of neural code is something that communicates to other parts of the brain that we are uncertain about, but it is only one of many codes. You could, for example, say that there's just a cell that says,"How uncertain is my visual system at the moment?" in which case, everything that has uncertainty has no effect whatsoever. No messiness involved, ever. We basically have one cell that basically says, very uncertain, not very uncertain.
Matt:Interesting. So if you get an ambiguous sensory input and you're trying to do object identification, you could, once you match it with the best thing, you could just snap. That's it. You've made the decision, right? You've sort of applied your vision of a guitar. If you've decided that's a guitar, you make the decision, I'm going to apply my idea of what a guitar is to that object in space. Right? And it's no longer really you're certain about. I mean your certainty, at least, has gone way up because you've made that decision.
Konrad:Right. Let me show you two areas in which case these two ways of thinking about uncertainty feel very different. So let's say one case where you see a guitar and it's very dark, so there's a small number of photons so you can't be sure if it's the guitar. And in that case, you can say maybe the neural activity is very massive because kind of stuff comes in and we're unsure how to interpret it and that's less, a small number of photons. In that case, like this disorderliness seems like a very natural way of thinking, but uncertainty. Let me take you to another case, where it seems like really weird. So I studied movement a lot. So in movement, when I don't show you your hands, you don't see your hand for a while, it turns out that you become uncertain of where your hand is because your proprioception has this thing that's called"drift." So basically if I rotate your hand here and hold it long enough, you will kind of no longer know exactly where it is.
Matt:I know exactly what this feels like because I meditate, and sometimes I'll wake up feeling like my arms are in a different place knowing that they aren't really there.
Konrad:That is so cool!
Matt:But having that sensation that they are somewhere different.
Konrad:There's also the, do you know the rubber hand illusion?
Matt:No. What's that?
Konrad:Okay. Rubber hand illusion is a myth. So they take a rubber arm that's not yours, they put it next to your arm, then they hide your actual arm. Okay, so there's like now a divider. Think about it like there's a divider, you see the rubber hand, you don't see your real hand, and then what they do is they stimulate the rubber hand and your real hand with just some mechanical device at the same time. And after they've done it often enough, it feels as if this rubber hand is totally your hands.
Matt:Yeah, that makes sense to me because you're sort of like projecting your experience that you've several times predicting that you're going to feel this and since you see it happening, even though you don't feel it happening, I'm like, well that's good enough. That's good enough.
Konrad:But you feel it happen, that's how they trick your brain into it, by stimulating it at the same time. Whenever you see that the rubber hand gets touched, your real hand gets touched. And by doing that often enough, they convince your brain that the rubber hand's totally your arm. And then if someone gets out a knife and stabs into the rubber hand, you will be scared to death.
Matt:Right.
Konrad:So that's the rubber hand illusion, but what it shows is that you have considerable uncertainty about where your hand is now when you don't see it. Now, here's the interesting thing. If you want to know how much uncertainty you have about where your hand this, it's not something fuzzy about the visual input or something. It is about you memorizing how long it's been since you last saw your hands.
Matt:Oh right, right.
Konrad:Okay. So in that case, how uncertain you are of something is not something that happens from the visual stimulus. It's exactly the same as your stimulus. It is something that comes from something that–
Matt:Temporal sensations over time, right?
Konrad:That's right. It's something that you need to learn about. Something you need to integrate.
Matt:Your uncertainty should be based on what you're doing at any moment in the context of your actions.
Konrad:That's right. So in one case, uncertainty something that's in the image. In the other case, it's something that you learned over time. It is not in the image at all, but it's something that's instantaneous.
Matt:Right.
Konrad:So in that second view, the idea that uncertainty is sort of something that's in the fuzziness of the neural representation kind of doesn't make much sense because the stimulus exactly the same. Where is that uncertainty at that part coming from?
Matt:Some other disconnection up here.
Konrad:Yeah. No, this is weird.
Matt:Yeah. I mean you can convince– this is sort of the problem with belief. You know, you can convince yourself over time if you're given enough evidence, that's something completely wrong is true. You know, and you believe that until you get enough counter evidence that you can change your beliefs. And if someone convinces you experimentally, that's your hand, that's your hand, that's your hand. I can see that they're tricking you. They're changing your belief structure in your brain for that small period of time at least.
Konrad:That's right. And you cannot, of course, make a Bayesian argument for that. Like if the rubber hand actually is your hand, it is very unsurprising that whenever the rubber hand gets stimulated, you feel it.
Matt:Right.
Konrad:If the rubber hand and your hand would be different, how improbable is it that they always get stimulated at the same time? In a way, from a statistical perspective, your brain does the right thing. It's very likely that the rubber arm actually is yours, if you don't know that the experimentalists actually designs the stimulation to trick you into believing it's the same.
Matt:Right? Yeah. That is interesting. So we started off talking about– sometimes you're not able to find what you're looking for. Sometimes just looking for something you find a correlation that might not be the correct correlation because you're not looking at it from a more general aspect. So I think you've written a bit about how to do these type of generalization studies. Is that a way to counter this, in experiments anyway?
Konrad:Yes. In experiments I think in neuroscience, we really need to stop doing generalizations studies much more. So what do we typically do is we do one experiment and then we have one theory that goes with that experiment. In a generalization study, I do one experiment, figure out what the theory is for that, and then I do a very different experiment and see if my theory still works. This is something that we almost never do in neuroscience and rarely in psychology. These resulting theories from it, they are not put to the test. If you, for example say, here's the brain area, we recorded from it. We find that nuance have tuning to orientation. Therefore, this must be an area that does orientation discrimination.
Matt:Hubel and Wiesel stuff.
Konrad:Hubel and Wiesel stuff. And a lot of– and it's the same logic of a lot of what came afterwards. That statement without a generalization study means almost nothing for all that we care, but every feature of the world that we could change, could change the activity of those neurons. There was a recent Carandini study that basically showed that locomotor activity is all over the visual cortex. So without a generalization study, it's very hard to know what we have learned in theory space.
Matt:So how should neuroscientists go about that? Well, one of the things you do is you collect data from a lot of different places, right? You create your own studies because everyone's got so much data. So you think that there's an area that's ripe for other labs to try and do sort of these cross generalization studies using existing data?
Konrad:Yeah, I think times are pretty good for us data parasites.
Matt:Yeah, a lot better than five years ago, I'll tell ya.
Konrad:And like I very much believe in there being an ecosystem for ideas. Like what is the probability that an experimental lab can look at the data and figure out statements that make sense about intelligence? Like that glue between data and ideas, that is something that we have in the past often delegated to purely experimental labs, and intelligence is pretty complicated, at least as far as I'm concerned for the moment. I think we need an ecosystem where people can come up with ideas, find ways to formalize it, find ways of testing it, and that used to be very difficult. And it's not just on the experimental side, the same thing on theories side– that lots of theorists loath actually getting their hands dirty and show that their ideas are born out in data.
Matt:Right.
Konrad:I think we need those bridges.
Matt:Well, I'm totally on board with that cause. I think that there's so many good ideas out there and so many people approaching this problem from different places. Making those bridges is super important so that we can all build something and progress this area together in the future. So that's the end of part one, folks. You can continue on listening to part two of this interview with Dr. Konrad Kording, when it comes out in February. Look forward to the next Numenta On Intelligence podcast. There'll be another Interview with a Neuroscientist. Thanks for listening. I'm Matt Taylor from Numenta.