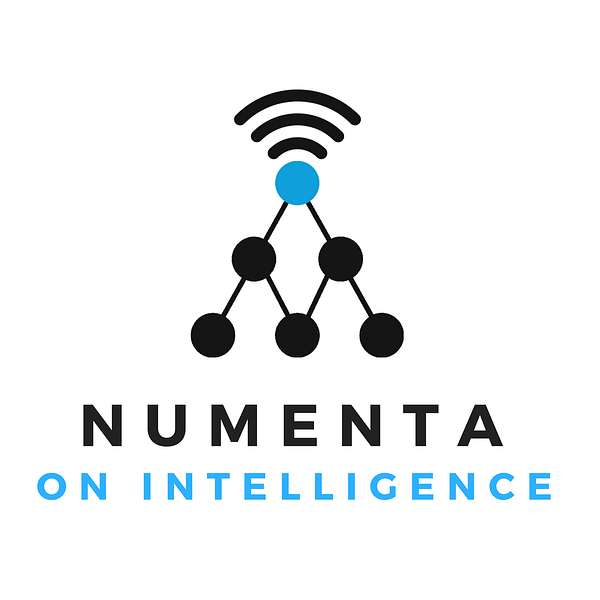
Numenta On Intelligence
Numenta On Intelligence
Episode 4: Natural Language Understanding with Cortical.io's Francisco Webber
Podcast host Christy Maver interviews Francisco Webber, CEO and Co-founder of Cortical.io. Cortical.io is a strategic partner of Numenta that specializes in natural language understanding. In this episode, Francisco talks about the spark that started it all for him while watching a YouTube video of our Co-founder, Jeff Hawkins, the advantages of their patented semantic folding methodology over other machine learning, statistical-based approaches, and the many natural language use cases the company addresses.
You're listening to Numenta On Intelligence, a monthly podcast about how intelligence works in the brain and how to implement it in non-biological systems. I'm Christy Maver and today I'll be talking with Francisco Webber, CEO and Co, founder of Cortical.io. Cortical.io is a strategic partner of Numenta that specializes in natural language understanding. In this episode, Francisco and I talk about the spark that started it all for him while watching a YouTube video of our co founder, Jeff Hawkins, and how their approach differs from other machine learning models. As a reminder, if you want to keep up with the latest Numenta news subscribe to our newsletter, which you'll find on our website, numenta.com, and you can follow us on all things social at Numenta. All right, hope you enjoy this conversation with Francisco Webber.
Christy:Hi, this is Christy Maver and you're listening to the Numenta On Intelligence podcast and I have a special guest here with me today. Francisco Webber, CEO and Co, founder of cortical Io, which is one of our strategic partners. Cortical.io is a biologically inspired natural language understanding company. So Francisco, thank you so much for joining me today.
Francisco:Thank you for having me.
Christy:Yes, absolutely. I think natural language processing and natural language understanding seems to be such a hot area of interest right now. And you guys are doing something really unique. So talk to us a little bit about Cortical.io and what you all are doing.
Francisco:Yeah. So, uh, in fact, uh, we are focusing very much in a going sort of deep into the understanding of language, um, because the situation that we're in is that we have, of course this a data explosion in general, uh, and what makes it worse and worse is that the balance of the data we need to work with goes more and more from the numerical transactional part, which we do already handle pretty well with database technologies and all these kinds of things. And more and more text data becomes the key asset in many businesses. For example, an insurance company. The only foundation of their business is text. So it's contracts, it's a description of things that the insurer and things like that. So the whole business is basically processing text on a very large scale and by becoming bigger and bigger companies have more and more customers that end up with huge amounts of text and the only way out of this for the moment at least is to hire a lot of people who read the text and then do something with it. And that's why this potential of streamlining business processes of getting them really efficient and really quick, very similar to what happens on the transactional side, we just need the tooling for that. And so the interest in natural language understanding was growing a lot in the recent time. But I can assure you we are struggling with this already like 30, 40 years intensively.
Christy:So your whole approach is that you're, you're doing natural language understanding based on the brain. Right? And obviously that's the tie in to Numenta. So what does that mean exactly what or how is it different than what most approaches are doing?
Francisco:So this is all about trying to be different in the first place. So in my previous, um, sort of project and endeavors, we were trying to apply just the state of the art in doing this kind of processing of text information, but it turned out after many years from me and many, many years for, for the photo scene if you want, um, that by applying this very same principle, we can achieve quick results. So we have managed to develop things like search engines and so on. Uh, but when you look closely, you see that the capabilities of these engines are like a 10 year old in general, is able to be more precise and more specific. Uh, then you could be with a, with a, with a huge computer computation cluster. The interesting thing is that although there was so much effort in research and so on, the improvement from what you get easily to how can I improve this, is so expensive that it seems that this whole effort we'll never actually reach levels that come close to what you could expect from a human.
Christy:And that's why people just hire many humans.
Francisco:Of course. I mean, there was always this part of the business, uh, environment where they try brute force methods to just throw in more money, more power, more anything. Um, but in that case it turns out that this doesn't really work out. Then you'll even see very large companies struggling basically. Like Facebook for example, currently struggling with just doing very basic functions of find me text where someone is talking badly or hate speech or things like that. Yeah. For a human I could probably ask my six year old son, um, to be a filter and he would perform better than any algorithm.
Christy:Is that because as humans, we are processing language based on meaning as opposed to a keyword or--
Francisco:Absolutely. I mean, it's all about the meaning. That's one thing. Yeah. Uh, it's all about how do I capture the meaning, how do I represent meaning? These are all big questions. I mean, one of my favorite topics is the representational problem, is a key problem in trying to get the computers be more like humans. On the other hand, uh, in, in sort of traditional research strategy if you don't yet understand the system that you want to study or that you observe, what you do is you record behavior of the system and you try to get as many as you can and you do a statistical model of what you have seen. And by having the statistical model you can then play around with it and try to figure out how does it actually work internally. With a system like language that has such a huge space that it covers in terms of content, in terms of variations, yeah, this seems to take forever. Yeah. Uh, and so, uh, the question was for us, how could we improve things substantially? And the only way to, to look for a solution was to drop this whole statistical modeling in the first place. But of course, what are you going to do?
Christy:Then what do you do?
Francisco:And, uh, so I, I keep saying the only known reference implementation for a well functioning language engine is the human brain. So the answer has to be there. Uh, and the brain is not an infinite structure. So it's-- the problem can't be infinite in the sense. Um, and uh, the fact that my personal training is a, was in medical school in Vienna, made this sort of natural connection of trying to see what has nature, uh, tried to overcome this. Um, and that was also a when, when I first crossed the work of, uh, of Jeff. It was a YouTube, a YouTube video of a talk he gave at Almaden, I think it was, and it was, in fact when talking about sparse distributed representations came up. That was the moment that was sort of the spark for me, uh, to see the connection between what he's doing and what could be useful in terms of text processing.
Christy:So can you talk a little bit about sparse distributed representations specifically? So some of our listeners might know that's a, that's a fundamental concept in, in what we're doing. And it's all about how the brain represents information. Right? So it sounds like that was kind of the launching point for you too. So can you, can you talk about sparse distributed representations from your point of view and what it means in language?
Francisco:You can start by imagining a, a very simple situation. You see a cat and you have a lot of contextual information, uh, so you know, what kinds of sounds a cat makes you know, how it feels when you touched it for and so on, and all of that is triggered in the moment when you see a cat or when you hear a cat, for example. You have this a 360 degree experience, or view of the cat. Even if the, you don't see, you just hear it. Uh, it might not look exactly the same, uh, but, uh, there is very little that the cat could do that would surprise you. You're prepared for a lot of aspects that are related to pets.
Christy:It wouldn't bark, it wouldn't...
Francisco:Yeah, exactly. So very obviously the way how a, a cat is represented in your brain is basically a sum of all these experiences. And in the end it's also a, there couldn't be anything else. I mean, everything your brain has is just recorded experiences. So whatever, uh, the brain wants to represent has to fundamentally be built out of components of these experiences which typically come from our senses and that's how we sort of naturally, uh, experience.
Christy:In moving through the world.
Francisco:Yes. So basically what happens is that when you see a cat, you hear a cat or in language, you read the word cat. Yeah. Then all these experiences of seeing, hearing, feeling, smelling, all the senses that are involved, um, uh, are evoked simultaneously. And this simultaneous, evocation of all these stored parts shows that the definition of how cat is represented is a distribution of many little events around cats.
Christy:Right and there's meaning embedded in each of those, as opposed to the brain saying, oh, cat equals 0, 1, 0, 1, 1...
Francisco:So, uh, when you hear, see, read the word cat, uh, everything in your brain basically triggers all the memories that are related to cats. This representation keeps using your whole brain. So it's in fact, at the first level, it's your sensory input. So you have images, mental images that come up or mental sounds, but it can also be secondary. It can, you can be remembering your mother talking about the cat in your childhood. It could, uh, be sort of a, a hierarchically stacked set of consequences that you've experienced around cats. So basically it uses all the different levels that are available in the brain. So basically it uses the whole cortex, uh, to represent everything. And this idea of using a vast space as a whole for basically every information that's there, that's what, what this sparse distributed representation basically defines. So when we compare that to a computer, for example, uh, if you want to store more information, you need to build up a, a long sequence of memory cells. And in each memory cell you put, uh, some data. If you have more data, you have to extend the number of memories you have. If you store in a memory that doesn't get bigger, where you always use the same space, you just change the pattern in which you store things. Uh, then it's very efficient. It's a very simple principle, uh, but to think it through, it's, it's astonishing how many complex aspects you will discover if you consider that to be the actual processing. And the other thing is of course, that this distributed representation also needs to be sparse so that you can differentiate between, uh, many different, uh, information states if you want. And, uh, we have found out in, in, in mathematically I would say, that there is a relationship between how much information you can store in this kind of memory and the degree of sparsity that you have. And uh, if the sparsity is not enough then you keep losing a lot of information constantly, and if it's, if it's sort of lower, you have more and more space to find different combinations.
Christy:Which is exactly how the brain processes information. At any given time, very few neurons are actually firing.
Francisco:And this distributed representation, just to give you an example, um, allows you to do something that in straightforward logic is not possible. Uh, it allows you to have a perception of something that two people have, which is per se different, which is this old problem. We all are different, but we, we are very similar and the same time know also how do you store something that is different and similar in the same time? Uh, so you have seen your cats, you have heard your cats and so on. And I have seen my cats, but still we can agree on what, for example, the word cat actually means even if we have different mental, actual mental representations. The trick is simply that because we are very similar, we have very similar bodies with two arms, two legs,
Christy:Same senses...
Francisco:Same senses and so on, there are still many experiences that we actually share and as long as your representation and my representation have sufficient overlap, we can agree on what a cat is. That's the simple principle that it's not about being equal in the representation, but by having sufficient overlap. So what you need is a mechanism that makes overlap the determinant for what is actually stored there.
Christy:So your representation of cat might involve a memory of your mom? Mine might not, but that doesn't matter that those two pieces are different because the core concepts overlap.
Francisco:Exactly, exactly. We have sufficient overlap. It's interesting because when you have a sparse representation, that overlap can be in absolute counting, pretty small or astonishingly small, and it can still trigger sort of a common experiences and the ability to exchange thoughts on this topic.
Christy:So you basically have have come up with a way to represent language where you're representing it based on meaning.
Francisco:Exactly. So what we did is basically we did a reverse processing, so obviously we humans interact with each other by having our cortices to exchange information. And in order to do this we have learned a way of encoding the state of the cortex and the encoding is called language. Yeah. So that's basically like computers have an encoding to communicate over the Internet. We have a, a sort of network layer that is carried by language and the way how this encoding happens is of course intrinsically related to how the brain actually works. If your brain works on transistors, then you're encoding has to be based on something that transistors can do. And the same for us. We can only encode using a method that our transistors, if you want, can actually do. And that's precisely what comes out of the work of Jeff. He described the sparse distributed representation, for me at least, as a set of constraints. That was basically the starting point and we went back from the language and we said, okay, which must have been the processing step to end up with this representation. Um, and that is basically what semantic folding describes.
Christy:And semantic folding is really what Cortical.io has, has come up with.
Francisco:Yeah, it's basically using a lot of concepts that we know in computer science already for quite some time, but it uses them in a new combination or a new setup. So we haven't invented new, uh, I don't know transistors, but we are using transistors in a different way than we have been using so far.
Christy:One of the examples that I, that I like and you, you actually have a lot of great demos on your website where people can go in and play with.
Francisco:Yes, to experience what that actually means.
Christy:Yes, because essentially you're, you're making language computable, right? Based on meaning. So, um, so one of the examples that I like is if, if I say the word apple to you, especially sitting here in Silicon Valley, you don't know if I'm talking about the fruit or the company down the street, right? And the brain can hold both of those representations at the same time, which means all of the things that I know about apple, the fruit and all of the things I know about Apple, the company are firing. But then if I say apple and I pull out a red delicious, then your Steve Jobs neuron is not going to fire.
Francisco:Language is the way it is because of our brains. So were our brains a bit different, the language structure would be different. And for example, one of the reasons why nearly every language has something like a sentence is basically to create a context for each word in the sentence, to allow you to effortlessly disambiguate. If, for example, I use the word smell together with the word apple, uh, within, uh, a fraction of a second, any Steve Jobs thinking would go away because that is not probable to be related. It's much more probable that the apple falls from the tree has something to do with smell.
Christy:Yes.
Francisco:Uh, it's even, uh, on a, on a sort of a linguistic level, you can observe similar phenomenons. Uh, again, with the example apples, apple, uh, if you make the plural, if you put an s in the end, immediately, there is no computer coming up in your mind.
Christy:There's no company.
Francisco:So it just to say that for example, to, to, to show you the difference to the standard approach, uh, with statistical natural language processing, because you are confronted with this huge combinatorial space, you have to make your model simpler because otherwise the computer never ends computing. With statistical approaches we very often discard that information. So we say in order not to end up with too many words, let's cut down all the apple, apple's and so on to some common root, and then you lose that information. And by losing the distinction of plural or singular, it makes the disambiguation even harder. Uh, and that is the reason why you have this quality ceiling in a statistical model because you just throw away a lot of important information in order to make it computable. But if you would find a way, uh, that actually uses all these aspects and makes them computable, then we could try and go further. And, and SDRs, so sparse distributed representations, are actually the solution to this. Sparse distributed representation, especially for a German speaker, is tough to pronounce. Um, uh, we, uh, decided to call this a semantic fingerprint. It's also sort of, it also, I think better describes on how we used it. So depending on where you are in this, in this area, that the semantic fingerprint covers, uh, the bits that you find there stand for different contexts, semantically, different contexts. So you might have all the sort of a different sounds the cat makes in one area of the fingerprint, uh, the different, uh, types of fur that the cat could have on another area and looking at the whole gives you the representation of what cat means or what cat could mean for.
Christy:Okay. And we'll, we'll put links in the show notes, but just to give a visual for people that are listening when a semantic fingerprint is essentially a matrix of how many, how many cells?
Francisco:So we currently, uh, so in nature that must be huge, millions by millions. Um, uh, in our technical implementation, we have found out that, uh, an extent of about 128 x 128 possible positions, uh, is a very useful sort of size. But theoretically you could choose any. It's a deliberate choice on how big you want to have it. Okay. Uh, so we use for standard language, this 128 x 128 gives you 16,000 bits, every bit being a feature which means a semantic context. So at every location of my 2D extent, it's like a little square with those 16,000 dots in it. Um, every position stands for different topics and the arrangement, and that is crucial for being practically useful, is generated automatically without needing any human input in the first place. So it's unsupervised basically.
Christy:Okay. So I could have a semantic fingerprint for the word cat where each each bit represents my experience of a cat or I could have a fingerprint representing a document where each bit represents topics of the document is or it could be a sentence, right?
Francisco:Yes. And, and an important point is, and that's also a sort of a differentiator to, to the state of the art, uh, in order to generate this topology and those contexts, we don't use the data that we actually want to process because the big problem in AI is that we always hit a ceiling, um, that is typically called,"to solve this, we need world knowledge." So in order to solve certain things, especially if you come close to what humans do, uh, there has to be some prior. And the big question was always okay, how to bring this prior in to use it to describe what the actual data shows. So what we humans do is we go to school, we go to university, if I want to become a medical doctor, a I read books and I listened to medical doctors speak and I learn the language and the thinking based on the language for that domain. And once I have done this, then I can work in the hospital and I can start reading patient records and understanding what is meant there. But it's not by reading 300,000 patient records that I become a doctor. And in order to bring in this prior world knowledge that is needed to have a, what we call a semantically grounded information, we have to bring in training material that is not actually the material I want to work on. Um, and this might sound complicated, but in fact, it makes it easier because reference material is something we systematically produced as human civilization since very long time for one reason, because we need to teach the next generation the findings that we have done so far. And this has been refined to the degree that you nowadays have, I don't know, a couple of hundred, probably key publishers who are, whose job it is to gather this reference information to build it up in an adequate way. So interestingly, it has to be built up in a way so that humans have it so that it becomes easy for humans to build up that map in their brain about that topic. And if you look carefully, you see that an author who tries to write a textbook, carefully structures the text. He makes titles, paragraph sections, uh, he puts certain words, bold or italic. And so in all these aspects are in fact an encoding mechanism for capturing this whole ontological systematics that you find in basically every domain. Okay? And when we try to extract this, we say one context is a sentence because a sentence stands for a certain fact, but it's also a sentence that appears under a certain title. So we add the title to the sentence and the title, uh, is under a certain section that is also titled. So we take the whole sequence of titles with the actual sentence and all of that becomes one specific context and the context stands for all the words that are actually involved. And so there you see that, uh, the whole treatment of the training data already tries to be intelligent instead of having sort of the brute force approach where we say we don't care what the actual structure of the data is, if it's, if it isn't enough, we just take more of it, which is the statistical approach.
Christy:Yes.
Francisco:But it shows that by just trying to be a little smart, you can be so much more efficient that for many cases, uh, we could, uh, create a semantic maps in domains where there hasn't been any machine learning so far because there isn't enough material.
Christy:Wow. So I want you to talk about some of the use cases that you're, you're, engaged on.
Francisco:So fundamentally what every solution does that we create for our customers, is first we define the semantic space that the customer works in. So if the customer, for example, happens to be a, a, a bank. So we have a couple of, of banks, customers, um, their professional language is let's say a English investment bankerish, let's call it. So we try to find typical literature that people who end up in that position learn. So we take financial textbooks, uh, textbooks about the investments and so on, uh, and by ingesting that, we define the kind of language that these documents, for example, are written in. And based on that semantic space, which we call a, it's a bit ambiguous, but we call it Retina.
Christy:Retina
Francisco:This is historical because we always say that it is like looking at the words, um, and this specific Retina is now used to convert any given document in that domain into a fingerprint, into this sparse distributed representation. And the goal of it is that if I have any two fingerprints, I can measure how similar the text is that they were generated from. So let's imagine you have a phrase that says done deal, um, and you have another phrase that says, signed contract. So any average banking person would say, okay, these are similar.
Christy:These mean the same thing.
Francisco:They don't use the same words, right? Um, and our Retina in fact converts both of them into fingerprints where you have an overlap of let's say 35 percent, which is typically saying they are very similar. Okay? And the nice thing from the fact that we have this thing, this two dimensional fingerprint representation, is that we not only can count how many common bits, how many bits are set at the same position in both of the finger fingerprints, but also where is this overlap? Because I do have this topology so I can inspect it. And I can find that there is a region where the two overlap and the context behind it are about investment, money transfers, legal aspects and things like that which are typical for the context of"done deal" and"signed contract." And the interesting thing is that making two pieces of texts comparable is the atomic function out of which you can build everything merely in language. So if I can compare two documents, for example, I can start searching through document collections because if I have 100 million contracts, I want to make them searchable. I just convert each of the contracts into a semantic fingerprint. I allow you to type in what you are interested in, literally typing in a, I would be interested in contracts that are about whatever and then make a fingerprint of what your description of what you are looking for. Then I just need to see which of the documents has the biggest overlap with the fingerprint of the query of the user.
Christy:Rather than having X many humans either comb through text
Francisco:Read or by matching what we do now in search engines, by matching the keywords. What words have you been using for your query and what other documents where these words appear?
Christy:But in the example you just gave, the words didn't overlap at all, so that wouldn't help.
Francisco:So that's precisely the problem. So if the words that you used for your career appearing the text, yes, chances are high that the text is relevant to you, but that doesn't mean that only these texts are relevant to you. There might be much more relevant documents, uh, but they just don't use the same wording. This problem is in fact a very frequent because very often you have lesser skilled people search for information that was written by more skilled people. So there is an incompatibility of the language. So you are missing a lot of information by default. That's a, a sort of typical building block in our solutions is the ability to do semantic search.
Speaker 2:And a semantic search in general can be defined as a mechanism that allows you to find information or documents, for example, that do not necessarily contain a word from the query. And this mechanism itself can again be, sort of used in many different environments. So, uh, banks might want to search through large collections of contracts. Um, pharmaceutical companies might want to search through collections of scientific publications for doing exploration, finding new molecules, or car producers might want to index their car manuals to allow you while you sit in the car to find out what that light means or how that function could be. I mean, a modern car so complex that you end up having a handbook of like 1,500 pages. And we have actually made a use case with a car producer in Germany and there, the case of the incompatibility of language is very evident because car user is an average person and the car manual was written by experts about cars. And so we created a system that was trained on automotive engineering concepts as well as a chat about cars by private persons, by aficionados, sort of. Yeah. And we use both, um, um, language components and mixed them in one system. And in the end, you could search through the handbook by, for example, typing in"where's the donut?" and it would put you on the page where the spare wheel is actually packed in the car, basically to demonstrate, uh, the meaning of a word that in principle has nothing to do with cars, for people who know about cars, could be mapped to the actual term and concepts that you want. So, yeah, this is a typical
Christy:So lots of different use cases across different industries.
Francisco:That is in, in, in business terms, this is one of the challenges, in fact, uh, that that's a technology that is so horizontal.
Christy:Well every business has text.
Francisco:And that is also, again, because, uh, we are used to always differentiate us from a, a state of the art, uh, approaches. So a typical machine learning approach in solving a business problem is to try and, and get more and more and more and more specific until the brute force model does a good job. Uh, so you can use machine learning to solve the business problel of discriminating between cats and dogs. Uh, but if you want a for another customer to discriminate between Lions and tigers, uh, you need to rebuild the whole model and create the whole effort once again, and if others want to have the difference between Indian and African elephants, it's yet another model again. In our approach, once we have generated the semantic space, let's say about a investment bankerish, nearly all the use cases that we find in the domain can use this, uh, this semantic space. So for the customer, it's a very efficient way of consolidating systems so they can start, for example, to build a semantic space about the products that they sell a for the marketing department. So they, uh, in order to represent marketing information properly and so on. Uh, and with the same semantic space, uh, they could then go and start a support system to help the customer, the customer care in the, in the call center to find the support documents easier because it's the same language, it's the same products. Um, and, uh, and, and that is sort of one of the business advantages of our approach is that you can build up a, a system that reuses everything that has been built so far, even if it's in a completely different use case.
Christy:I should mention Cortical.io has been a strategic partner of Numenta's for three, three plus years now. And it's really great to see the evolution of how you're applying this in so many different ways.
Francisco:We are still in its very infancy, uh, because, uh, what I actually want to do is to get to the point that we not only capture the semantics of things by using fingerprints, but that we also start to learn about grammar of things, which is defined by the sequence of those fingerprints went, uh, when I, uh, uh, take the word sequence, immediately one thinks of course of the sequence learner that, that we have with the HTM system. So the idea is to take the next step and not only to take, um, the lexical semantics. That's the level on which we work now. And it's astonishing how much you can do by just properly understanding this, uh, but the next level would be to take the sequence into account and then we can also handle language aspects that purely depend on grammar. Uh, so I do think that by applying HTMs, uh, on, uh, our semantic fingerprints, there is a lot of potential to, uh, get things like machine translation, like speech to text conversion. All of these things I think can be substantially brought to the next level, but there's a lot of research still to go.
Christy:Right. So the story continues.
Francisco:Absolutely.
Christy:So where can people go if they want to find more? We'll put a link to your website.
Francisco:Yeah. So we try to have a lot of content on our website. Um, so there is a lot to read there. There are references, there are lectures from people who speak about important aspects besides Numenta people of course, interactive demos where you can actually play with words, um, and, and get a feeling of what it means.
Christy:I've spent quite a bit of time on those demos.
Francisco:We do also have, uh, the, the functionality as it is right now, accessible on a public API, so if you want to have programmatic access to the functionality, you can request a, a key to use our API for free and you can also do more complex programmatic experiments. And we regularly get email and communication from people when they manage to solve tricky problems using our standard, even not specifically trained, uh, um, a semantic space that we offer there.
Christy:Nice. And you're based in Vienna, Austria, but you also have offices here in San Francisco and in New York,
Speaker 2:New York. Yeah. So currently besides Europe, the US is of course our biggest market. That's the reason why we have started the offices here. Um, and that's also what makes me travel a lot as you can imagine.
Christy:Well, we're always happy to see you and I'm particularly happy that you stopped by today. So thank you so much for your time, Francisco.
Francisco:Thank you for having me here.
Christy:And thanks for listening.