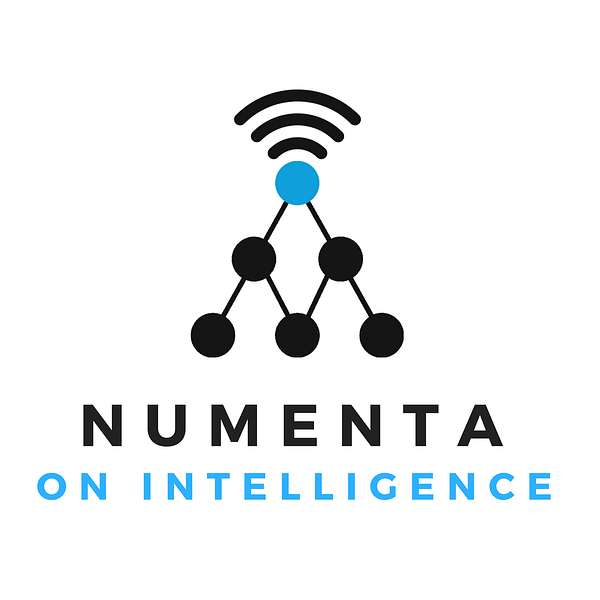
Numenta On Intelligence
Numenta On Intelligence
Episode 14: Interview with Florian Fiebig on Hebbian Learning Networks
Matt Taylor interviews Florian Fiebig, PhD, a Visiting Scientist at Numenta.
Florian Fiebig is a research scientist at Numenta recently graduated from the KTH Royal Institute of technology in Stockholm, Sweden with a PhD in computational neuroscience. He's been sharing with us his work on Hebbian memory networks which he describes in his PhD thesis linked in the show description. He's recently been featured in our research live streams, talking about synapses and plasticity. You can find upcoming live streams featuring Florian by subscribing to the Numenta YouTube channel. His thesis not only provides new memory theory but also provides a general introduction to memory and learning in cortex. It is called active memory processing on a multiple time scales in simulated critical networks with Hebbian plasticity. I won't make you remember that. You can find a link for it in the show notes where you can download a PDF for free. Thanks for listening to the Numenta On Intelligence podcast and I hope you enjoy this interview with a neuroscientist. First of all, welcome to Numenta. It's a pleasure having you here.
Florian:It's a pleasure to be here.
Matt:I have been spending a good amount of time reading your PhD thesis and the three papers in the back. A lot of the things that I'd like to ask you about come from this thesis. For those listening, it's called active memory processing on multiple timescales and simulated cortical networks with Hebbian plasticity.
Florian:That's a mouthful.
Matt:And we'll put a link in the show notes so you guys can, it's a freely accessible in PDF format, which is great b ecause it's really good. Honestly, it's a great introduction to memory frameworks in general. I think in neuroscience, especially Hebbian memory, which we'll talk about i n[ inaudible],
Florian:I wrote, like in part it's a lot bigger than a dissertation has to be just because I wanted my family and friends to be able to read some of it and not just my colleagues. Uh, so
Matt:was super nice of you to do that. Cause I mean it opens the doors for so many other people because I mean you go to, I mean I'm not a neuroscientist. I've been reading neuroscience papers for a few years now and some of them are just like immediately a brick wall that you can't get past because I got to look up that. I gotta look at that. I go to[inaudible].
Florian:Well, the good thing is just like, just like Jeff, I've gone through this experience of sort of coming from a different field before and then falling madly in love with neuroscience and then, you know, like squeaking your way into the, into the field. And so I've had this experience for like one year, you know, dedicated, trying to push into neuroscience and tried to learn about neuroanatomy and electrophysiology and whatnot.
Matt:Well, maybe you can talk about that, your background and what led you to Numenta now?
Florian:Yeah, my background is, I'm an engineer, so I studied, uh, the technical university, Humboldt uh, general engineering science, um, pretty much every branch of engineering that is just because I couldn't get enough of it. So chemical engineering, electrical engineering process, engineering, mechanical systems center, uh...
Matt:You migrated to the really big questions.
Florian:Yeah, little stuff. Like I had a lot of site interests and I had a hard time making up my mind. So like, you know, patent law on basic economics, whatnot on the side. Uh, but so eventually I got interested in process optimization, uh, which also led me then into, um, AI and robotics. And so I found this really cool master program in Stockholm, which was quite a modular, sort of appealed to my, uh, sense of navigating enough, being able to elect any courses that I would like because systems control and robotics, that's kind of everything. And then I specialize in AI and machine learning and getting increasingly frustrated with many of the, um,[inaudible] a little bit with, um, not the simplifications per se. I like simplified models, but the refusal to look at the brain for an inspiration. Um, and so, um, I was aware of Jeff Hawkins and his work for quite some time and so I eventually figured out if I'm going to do this, which I'm going to have to do it myself. And so I just sort of retrained myself like on the side in neuroscience and started sneaking into neuroscience and extras and asking people in the, in the back row for the password for the course webpage. Wow. Wow. Like seriously? Yeah. Anybody can not anything if you even want to. It's, I mean you don't need anybody's permission to learn something.
Matt:I mean your doctorate's in neuroscience, right?
Florian:Yeah. Oh, mine is computational neuroscience. But of course that's a, that's a branch of neurosciences, right?
Matt:Were you bestowed a sword when you were giving your PhD, cause I heard in Finland anyway, you get a sword when you've become a doctor.
Florian:No, no sword. Uh, I got a bunch of like, you know, heavy rocks of sorts. Um, like, um, they're like artistic things. One of them was actually very nice and it's like this inset stone into a glass sculpture of a head. So it looks like you're peering inside the brain. Ooh. Um, I forgot the name of the artist, but it's actually quite beautiful and you get this doctor student, I still should do like a proper like ceremony, like a PhD, you know, graduation ceremony. There was one now or that I missed of course, cause I went here. Uh, but you know, they don't take that away from you. You can go whenever I get a ticket. And technically my PhD is also dual degrees, so I get one from the university of Edinburgh as well and they get a kilt. So that's one more reason to show up at another and not to, to get myself a proper kilt.
Matt:Excellent. Oh, well speaking of your, your doctorate thesis, so I haven't read all of this, but I read enough to think several times this guy should be working for us. This is, so many times I was like, that's exactly how we think the brain should, should work. So I love that you're here and let's talk about some of this Hebbian learning stuff. So we're going to get into some of the nitty gritty of neuroscience and this, this is a podcast about neuroscience. So let's, so let's do this. Let's talk about Hebbian learning. One of the things that the two factors in Hebbian learning, right? There's a, there's a learning rule that that requires a pre, why don't you talk about that first?
Florian:Uh, so I mean we can go back all the way to sort of this a simplified version of what[inaudible] had actually said. Like you know that fire together wire together. It's not what he actually wrote, but it's what everyone says. That's what everybody says he wrote. Right? Um, but the, the, the point is that, um, fire together obviously means, um, that you need to have some metric, some measurement by which you can observe whether they are actually active together. And so since there are two neurons, um, you always have what is called the pre- synapse and the post synapse. So, um, and so you need to track activity on two sides of the equation. And then you also want to measure, uh, how many times the active together, right? So you want some, some. Um, so essentially you have three measurements, right? Um, the, the, the presynaptic spiking, the post synaptic spiking, and then what your peculiarly interested in in Hebbian learning is, um, the, the correlation between the two.
Matt:How closely together and time, right?
Florian:How closely in time and how closely are together in intensity and like different learning rules capture different aspects of that. Right. Um, and of course, uh, it matters a lot because there some learning rules have a tight temporal kernels like STP, uh, spike time dependent plasticity kernels, um, tend to be very narrow. So like if there's spikes on the pre and the post synaptic site don't occur within like, you know, 10, 20 milliseconds, then there won't be much of a synaptic change. But many of the protocols really using for inducing lasting associated change in neurons where we, you know, bombard neurons with long tetany of pulses, uh, which are not necessarily biological, but very effective. And potentiating synapses making them strong, right? During experimentation. Right. So like you have a, you have neurons that you've grown in addition, there's a synapse between them and now you want to investigate how the synapse changes with, with activity. And so, um, then we do protocols that are a lot better at capturing sort of rate relationships rather than the individual spike time. And so that's why it also matters to understand that there's so many different forms of plasticity.
Matt:So if you're thinking about a rate, you're going to look at it over a time window,
Florian:But you're averaging, that can be like an exponential average or like some shifting time window.
Matt:So there's a lot of ways you can apply heavy and learning. Sounds like you could do it one spike at a time and have a very simple rule or you could have some oscillatory rule.
Speaker 2:So quite a quite a zoo out then. The interesting realization is of course it's not just the zoo in terms of mathematical models that sort of abide by this broad idea of Hebbian learning, but there's somehow capturing correlations. But there's also a zoo of bio molecular mechanisms that support that, those, uh, those ideas. Um, there's many forms of plasticity Hebbian learning, obviously just one of them. Um, but there's several of, you know, uh, possible mechanisms that could implement something like that in the brain.
Matt:Is there, uh, does, does deep learning have anything similar to Hebbian learning like a two factor rule? Like we're talking about at all?
Florian:Oh, that's a good question. Um, well, I mean, like gradient descent per se doesn't really do that.
Matt:You need some type of localized feedback, right?
Florian:Yeah. Because of course, I mean the, that that is exactly right. I mean, it had been a local rule because all the information that the synapse has is only the information on the pre and the post synapse and we think of them as separate, but in fact, there's a lot of, u h, messaging between them, not just the, you know, pre-synapse, u m, sort of releasing neurotransmitter that then gets detected and l eads t o a change on the other side. But there's also a retrograde signaling and sort of, there's a lot of communication, but i t's all very local, just a small volume so you don't get these big, a full network kind of u h, analysis things to kind of reshape the whole network. You k ind o nly d o i t from the smallest element u p and see what emerges when you c hange t he local rule.
Matt:So everything's locally learning simultaneously. That's sort of the emergent thing.
Florian:That's right. And that's what's makes it a little bit hard to wrap your head around because we are so used to designing systems top down rather than bottom up. So that's why also many of these things require, you know, building things small and then testing how they actually behave when they grow bigger because we might not be able to predict a priori sometimes with a very simple network, you can predict these things. You know what the distributions of weights for example, are going to look like a certain conditions. And you know, what kind of, you know, dynamical structures kind of get formed or not. But as soon as you go have any amount of biological detail you get so many non-linearities and u h, u h, a nd you know, so many differential equations that interact with i t, with, u h, with another that, u h, it gets impossible to predict much. So that's why all of my work is very heavy simulation science.
Matt:Now, you hinted earlier that there were different types of plasticity in Hebbian learning. There's a fast plasticity and then there's a slower plasticity. Is it, is that synonymous with short term plasticity and what you might call longterm potentiation is it the right term?
Florian:Yeah. So a longterm potentiation is obviously a super important word in neuroscience, uh, often abbreviated LTP. Um, and so because neuroscientists have been asking this question, what does it take to change a synapse? You know, in a lasting way. And like, how do we learn? We learn things, you know, that stick with us just to buffer the last three words that were just said, right? Uh, but you know, how do I remember, you know, the name of my grandfather or something? Um, and so, so, uh, so it turns out there's very good experimental, um, sort of findings, uh, protocols that have are very nice in predicting how to potentiate synapses long term. The problem with many of those who said that require these, you know, long tetany, this long stimulus bursts at rates that are just outrageous for that for a real brain. Um, in part they require them because these are cell cultures, so they're taken out of the sort of InVivo context, right? So they don't exactly behave like the real thing. And also neuroscientists are impatient and they want reliable results. Um, and so, um, because of course in the real brain, you know, activity is a lot more messy and if there's any sort of burst activity tends to be very short. Um, and so as a consequence though, the most of the plasticity research early on was really does LTP research because it's relatively easy to do. Whereas these more fleeting forms of short term potentiation and there's a whole slew of them, some of them Hebbian and some of the non Hebbian. And in part my thesis makes the argument that particularly, Hebbian ones might be super useful for expanding things like working memory. Um, and so it feels to me like a lot of the research is still, you know, reasonably new, um, because it's not really, you use the term long term or short term, but the problem is that many of these uh, forms of plasticity are not really necessarily time dependent, they're more activity dependent, right?
Matt:Activity of the agent or activity of the cells around the neurons?
Florian:The pre and the post synapses. You can do get like a short term potentiation using very simple paradigms. But if there's any amount of ongoing activity, you know, just a couple of extra spikes, that potentiation will very quickly go away. Whereas if you then silence that cell culture or you cut all the inputs, go away for six hours and then you come back and then you test just with a presynaptic ping, right? You just send one spike through and detect what comes out on the other side. The synapse is still strong. So it turns out short term potentiation is not always short term potentiation. And in fact, the term short term is kind of misleading.
Matt:What is it? What should it be?
Florian:Yeah, I'm not sure yet to be honest. Um, because.
Matt:there's something else besides time.
Florian:Yeah. There's something else besides time. And we always model these dynamical systems which, um, with sort of some time constant. So there's a lot of temporal, uh, equations and that's all right. I mean, if there's a certain level of noisy activity, then if there's activity dependent decay or some signal then that will, you know, kind of get mapped onto time constant. But it kind of suggests that time itself is the driver for the, for the, you know, for the signals. And it might actually not be. And so it's a little bit misleading to always start these discussions about potentiation by saying, Oh, short term, intermediate term, long term, um, whereas in fact, you know, we might want to differentiate this.
Matt:Maybe the context is not so much time, but other biochemicals that are involved in it or some other form of context around the memory system?
Florian:So, yeah. Um, I mean I gave, um, two recent research talks now at Numenta about sort of fast forms of plasticity, which are not necessarily Hebbian, called facilitation and augmentation. And many of those are, are like reliant on calcium signals. Um, and it turns out that calcium diffuses relatively quickly and because of that there's a certain timescale to this phenomena and there's, there's iron pumps in the membranes that sort of restore the original level of calcium. And so that's why the signal will go away. Um, and so at least in the sense that the calcium originally drove it, of course these chemical cascades can get complicated. So it's not really just, you know, calcium, but it's often sort of the initiating mechanism of salt and calcium. And so, so then you really want to understand it's not really time dependent plasticity, it's more like calcium dependent plasticity.
Matt:That's more of the context. Usually calcium is there because of some time, something about time.
Florian:Yeah, you know, like a number of spikes that got transmitted over a short time. And so you had a lot of calcium influx.
Matt:A lot of this is just, um, trying to maintain some homeostasis in the cell or in or outside the cell. So there's biochemicals moving in and out just to get to a stable state.
Florian:Right, right. Homeostatic mechanisms are super important to keep the balance on many of these systems that otherwise would run away to weird indefensible states and I mean very rarely do we get a glimpse into that. Like in epilepsy when you have runaway excitation and there are these waves of activity that you'll squash over the brain and activate every neuron. And I often get asked by friends sort of, um, you know, what mom, just, you know, like how much of our brain is active and like this popular myth that we only use it a little part of our brain, right? Yeah. And then I tell them, look, I've, I've seen three times in my life, I've seen people with a hundred percent brain activity. It's not pretty to look at.
Matt:I bet
Florian:You have to be careful that these people don't swallow their tongue.
Matt:Yeah, it's not doing anything useful it seems. You know, I always think about this. Um, I used to, do you know what a Bobcat is? It's a, it's a, it's a small tractor, right? And it's got a scoop. So it's sort of like they call it a backhoe or a loader in the Midwest, but the controls you sit in it, it's just like one seat and it's like a small car. Right. And it's got a loader in the front and usually just move dirt or drill holes with it. So he's like, why is he talking about this? Well, okay, so the controls on it are two sticks. It's not a steering wheel. There's like a stick in your right hand a stick in your left hand. If you want to move the right track, you move the right one forward and you turn right. Yeah. So it was very much like a tank, cause you're on tracks or sometimes it's wheels. But um, so there's a state you can get in very easily. If you stop too abruptly, both of the sticks go forward and then you go forward to move back. And then you go forward and move back and forth, back and forward. The only way you can release from that is let go or else you're just in the loop forever. And it's really, really off-putting.
Florian:Interesting. That's such a great example of like viscerally experiencing.
Matt:Oh yeah.
Florian:Auto feedback.
Matt:Right. It's definitely a bit like an epileptic seizure, I guess.
Florian:You just get stuck in this, uh, in this, in this state.
Matt:Yeah. And all you can do is disengage. It's the only way to get out of it. And you only have to do that once and you learn it because it's such an easy way to get out of it. But you know, someone has to tell you Let go of the handles. And I remember that happening to me once. Anyway.
Florian:That's just a fascinating topic actually.
Matt:Let's talk about working memory, which a lot of your thesis is about working memory, short term memory versus longterm memory. So I found this fascinating cause you know, um, I, I've been thinking a lot about, um, where pathways, what pathways, you know, the different streams. And, uh, in your thesis you talk about short term memory and is it the medial prefrontal cortex somewhere around there?
Florian:Typically, I mean, frontal cortex in general is involved in all these top down things, but particularly for, um, sort of the kind of semantic declarative working memory maintenance and also lateral prefrontal cortex has been described to be i mportant. But that maps onto, if you map that onto a rodent, they don't have a[inaudible] o r prefrontal cortex f or, t hey talk about the medial prefrontal cortex. A nd so s ometimes it gets a little bit tricky when you're pulling studies from different animals.
Matt:Yeah. Well, I mean, mice don't ever have to make shopping lists or things like that. Cause that's what I always think about. I'm like working memory. I liked your paper because, u h, I don't how best to explain it, but you've got, u m, areas where you say longterm memory, maybe two areas of the brain that are different sensory modalities. Right. That are, that are performing longterm memory and your working memory is, u h, in p refrontal, u h, and that is sort of a scratch board or someplace that you can index, I like the word index there. So if I, if I go, this is what I do when I w ant t o see what I want to buy at the grocery store, I go to t he f rigerator and I s tart looking for things and I'll look for something and that will trigger, Oh, I also need milk i n addition to eggs and coffee and t hat sort of thing
Florian:Associative nature of much of memory.?
Matt:And, and so, so the, the longterm memory is getting sensory cues that identify objects, which then cues the short term memory. Uh, right? Maybe you could explain this better.
Florian:Yeah, no, no. We do this all the time. Like it turns out we have some of our working memory mechanisms are like really quite specialized for certain things. Like, so cognitive scientists have long described, for example, this, um, this auditory, uh, phonetic loop, I think it's called. So this idea that you can, even without much understanding, you can loop a phonetic sequence of some sort. So, um, rather than thinking of these five items that you want to buy and like visualizing them in front of your mind, you just speak the words. Yeah. Um, or, um, you build yourself, you know, like a little rhyme or something, buffering things. So butter, milk, egg, toast, and uh, I don't know. Cheese, butter, milk, egg, toast cheese, butter, milk, egg, toast, cheese. Like I can repeat butter, milk, egg, toast cheese faster than I can even think of these concepts and what they would look like in the packaging on this shelf in the supermarket. And so you can use that mechanism to sort of buffer that. And then you have these indexes, butter, right? Butter. Which b utter was it? That one. And y ou'll recall what it looks like because you have a representation. The longterm memory is all there, you know what butter is, right?
Matt:Yeah, you just need a trigger.
Florian:You need a retrieval mechanism. And so sometimes you can loop in these short term systems that are specialized. Um, you know, to very effectively get an index onto longterm memories. And that is of course an associative thing because you're binding these things together, right?
Matt:It seems like an extremely useful place to construct plans and goals and you know, things that you want to do, projects that you want to create. I mean, that's what the prefrontal cortex is all about.
Florian:yeah. All the executive and the planning and the imagination. Yeah, that's true. That's why these are all sort of like neighboring, um, functions there.
Matt:So, but, uh, in longterm memory we've got long term potentiation. Right? So the different type of learning. So in the short term area, would you call that fast Hebbian learning? A different type of...
Florian:yeah, that's typically how you would prescribe it. It's noteworthy though that that is a very new idea. Um, like the idea that um, longterm memory is associative has long been around has been shown in these LTP experiments that, you know, you get binding of co activated units and if you anti activate them in an anti correlated way, then they will actually uncouple. So like synaptic deep potentiation, synaptic depression, it's called LTD instead of LTP. Uh, that is long been known. But the idea for working memory was for for a long, long time that it would be some kind of persistent activity signal. So rather than having a system that buffers these items and some, um, synaptic changes, so people would think there's some kind of reverberating activity that self perpetuates somehow, it mapped very nicely onto early models of neural networks like these[inaudible] networks, which have these beautiful attractors so they can, once you let them once you kick them off, they can keep going for awhile. The problem is that you need dedicated attractor for all of these items and you can think of a billion different things. And if you d o, you get to the permutative complexity of all of these things you can think of. A nd then it very quickly becomes clear that you would much rather have a scratch board of some sort where i t can put things down and then gets quickly, gets erased. S o y ou don't have a dedicated network for all the working memory things you might want to keep s hort t erm on your mind. Even while you might have, you know, lasting attractors for sort of p rincipal things you've understood about the world.
Matt:Right. That's so interesting. I never, I've never thought about the prefrontal cortex as sort of being something that doesn't necessarily hold anything at the moment. It's just, it's used for like a, a desktop, you know, to put everything together.
Florian:Like these structures are very multidimensional. Um, and they're very, like these neurons might be doing one thing and one task and something completely different and a different task. Um, and so that suggests, um, that they're, you know, rather than university, they get recruited into things and fast, Hebbian mechanism would be one way to recruit them into some, doing something useful for some time. But because it's a fast mechanism, it also means it can be read to be quickly over written, but it might just be the temporal bridge that you need in order to kick off, you know, more lasting memory changes or just to perform the task. Right. Oftentimes we don't actually need the longterm memory. We just need be able to execute the task, which means retrieving some longterm memory thing, be able to do it, but then we can forget it. So we don't actually want to consolidate the full thing. You don't want to remember every word that I said today.
Matt:Right. There's no point in that. We forget almost everything we ever...
Florian:yeah, the vast majority I think of the brain as like this massive filter, right? So you have like all these impressions that you could perceive and a tiny percentage of that is even perceptible to the kinds of organs that we have. We only see certain wavelengths and we only hear certain frequency bands. Okay. And then out of all the things that you could perceive, only a tiny thing pass through your attentional gate. So, and so you're aware of even less than what you actually perceive. Clearly there's like, you know, pressure in my, I don't know, in my foot right now because it's inside a shoe, but I don't perceive it right. Unless I focus on it and then I notice, yeah, my foot has weight. Um, so, so you're not even aware of most of what you perceive and then even less of the things that you perceive actually become short term memory. So something that you are aware of for any amount of time, like some 10, 20 seconds. And you know, so I might remember, you know, the p ast sentence that I just used. U m, and so a tiny fraction of these things then b ecome something that y ou might remember tomorrow and o f all the things you remember from yesterday, you know, you're going to forget most of it. It's very unlikely that y ou k now much of that will be left after a decade f rom y our answer unless something extraordinary h appens.
Matt:And it's not necessarily that you'll remember, I hope these terms are right- the declarative experiences that happened to cause your semantic models to update. Right? You're just going to remember the facts that got updated in a lot of cases, it depends on the events that had happened.
Florian:I usually like to use this example of like, just try to remember, I don't know, like you might know that the capital of France is Paris, but do you know who told you?
Matt:No, of course not.
Florian:So one of the fascinating things is we have this episodic memory where we, you know, remember the exact event and what happened and this person walked in and said[inaudible] happened, but we also have this, you know, much more semantic memory and sort of things that stick around and things we learned to sort of deep connect from the context. And in fact, one of the most interesting discoveries that I made early on sort of that what I thought was super interesting about memory research was the fact that we have all these different systems and there don't seem to be connected very well in the sense that you can have one but not the other. Like you can, like, knowing how to ride a unicycle does not mean, um, you know, you might have a memory of when you learned how to ride the unicycle, but actually they're completely different systems and you can lose one without using the other.
Matt:Um, this is something they showed in H M.
Florian:yeah. H M this famous, um, famous case where they could teach him all kinds of interesting motor tasks, but you would never remember any, any of it in the episodic sense, right?
Matt:Right, he would get better.
Florian:Yeah. You could train them to be, you know, happy for something or to be afraid of something by fear conditioning or teach them certain modal tasks, you know, writing backwards in a mirror, things like that. Right? It's hard to learn, but you can't learn it. Um, but the interesting thing is, of course he would get better like everybody else because he had these systems that are necessary for learning these things, but he did not have the hippocampus, which is, you know, this super important bridge into a longterm episodic, uh, consolidation of memory. So you could leave the room, you know, I've come back two hours later and he would not even know that he had done that task and actually improved his performance on it.
Matt:Sure. That's fascinating. So we can get better at things without even realizing how we're getting better at it.
Florian:Yeah, exactly. Uh, and that also means that we need to get a little bit outside of like this story narrative we're always telling ourselves. We understand ourselves through what we remember episodically. But of course our learning is much bigger than that. Um, that is particularly obvious to all the kinds of learning that requires, uh, sleep and for all the kinds of memory learning that isn't declarative. So things you cannot state, right.
Matt:Things you can do, you have to do to learn them. Exactly. Performances.
Florian:Yeah. Maybe we should have like explained the term, right? So there's the text out of memory, our memory, so you can declare. So facts and events, so the semantic and what happened. Um, but then there's also all the non declarative memories, which are, you know, modal skills and uh, and, and fear conditioning. And, um, like all, all kinds of, uh, memories that you cannot state but you can still learn and acquire. Um, if some of those are like motor things like you can walk, right, but tell somebody how to walk. It's tricky.
Matt:You have to try it.
Florian:How do you, can you tell somebody how to ride a bicycle and then they will be able to do it?
Matt:Uh, no, I don't think so.
Florian:Probably not. They still need to practice
Matt:They've goe to do some trial and error. They don't know what it feels like to sit on a bicycle and to balance that thing. Um, yeah, that's,
Florian:so that's sort of a fundamental distinction. And the, obviously those are then associated with different brain areas. Um, and that's not that we don't use the other brain areas while we are learning those things, we're always using our whole of the brain. Right. But,
Matt:but those non declarative, uh, memories, I guess you could call them are very personal. It's like it's not, they're not facts and figures. They're tied directly to your body, your senses and the things that you do with them.
Florian:Which is why they don't transfer so well as words. Right. Whereas you can learn the capital of France. No problem. I can tell you. And then now I know this fact. Right. Excellent.
Matt:You mentioned this in your thesis and I find it fascinating because I'm, I'm a musician and I like to perform and practice music. And you noted that like a drummer, any drummer will tell you that, um, the rhythms that they learned that day aren't really gonna get locked in until they've had a good night's sleep and they come back the next day and then they'll pick it right up. And that's absolutely true. And I think any, any, uh, athlete or performance artists in any way, does it have to be a creative but an athletes the same thing. You, you have to have you to have that practice, that movement through space. You know, the update of all of your models and then get some rest, come back, try it again in a different context and then it sticks.
Florian:Yeah. And one of the fascinating things, so we can of course ask and that I like did much of my earlier work, uh, is how do these transfer processes work, right? How do, how does something go from this sort of initially acquired memory into something that is longer lasting? And so that's where all these interesting particular when it comes to, uh, like spatial memory, um, um, but also, uh, just episodic memory and in some sense, uh, there's this strong involvement of hippocampus, which we can read out and in rodents very nicely right. And you get all this interesting evidence of like this strong replay during sleep where whole episodes get compressed into like these short, like a hundred seconds short wave ripples. So you get these fast oscillations riding on a slower wave and they compress the whole movement sequence off the road. And that you saw before, you know, like behaving, you know, for 20 seconds or something, compress it down into this, this very short burst with the exact same neurons activating in the exact same sequence but not just once or twice, hundreds of times. And that obviously got people thinking is that causally related to their memory? And then people started meddling with it. So if you're not suppressed these, so you can have a microcontroller listening to those and then target to interrupt some of them but not others.
Matt:What is it that, uh, that, uh, tells your brain when you're asleep to take some events that happened during the day and reinforce them, but others don't worry so much about?
Florian:that's interesting, right? What about biases?
Matt:There must be some type of biochemical- dopamine is probably involved.
Florian:I mean, yeah, that's a big argument that, uh, that modelers have been making. I don't know how exactly strong, there's just sort of an interventionist sense whether you can prove that by like intermittently, you know, blocking[ inaudible] modulation of plasticity for example. U h, but that it's true that like the presence of dopamine at l ike these synapses i s, can, u m, can make the, u h, synapses a lot more plastic. Meaning they're stronger. And the hypothesis that my first paper makes is that the stronger synapses will then, u h, b e much more capable of reactivation. And because they reactivate, they get stronger. So t h ey're s o rt o f l i ke a s elf perpetuating dynamic.
Matt:They stand out sort of from the rest of the.
Florian:So the most powerful memories kind of run away. And so in that sense, the really question is if you want to remember something as we need two plans, right. Um, plan a is a just repeat it a lot. Right? Make it sort of strong by repetition, repetition. That's why y ou practice your, you know, vocabulary not just once or twice. Y ou go through your cards 50 times and that increases the odds that they will get consolidated. R ight? C ause it doesn't matter that, you know, now what matters is that, y ou k now, you know, in two weeks.
Matt:I like to change my context, gooutside and do something. I go over here and study the same thing.
Florian:And then there's of course the, the other strategy where instead of doing massive amounts repetitions, you make it really, really relevant.
Matt:Right, right, right.
Florian:Um, I dunno if you could have like outrageous example. Like you slap somebody in the face and tell, tell that person your name. They will never forget your name.
Matt:It's true. That's a good tactic to use.
Florian:Yeah. Right. Yeah. I don't recommend that I'm not a believer in violence. I'm very kind. But the point is we are very good at recognizing what is relevant and when something is relevant to us of much of that as obviously biologically, pre, pre coded. Right. So your pleasure and pain and uh, you know, anything with sex, you know, it's a lot better remembered. Um, no, this was just true. Anything that has social relevance on the stories involved and people, that's why all these memory artists, they map all their tasks, always onto, you know, people, places, locations, you know, things that we are all like automatically good at because we have dedicated circuits for that and things that mean something to us. They create like palace of the mind. You want to make these objects that you're putting in the space, like outrageous. You know, like you don't just want the chair in the corner, but know that the chair is like, you know, fiery red and like, I don't know, it's leg is on fire. Well no, you're not gonna forget that chair. It's a weird picture of one of your mind. But if that helps you build that palace of your mind for your memory task, that is in fact what they do with the more outrageous the story, the better our memory is.
Matt:Yeah. Okay. I mean there's, there's a reason for any performance artist or athlete that when they perform something well, it feels really good. Right? Because you get immediate feedback from that-you're, you're paying attention to the music you're playing or the ball that you're hitting or whatever. And when everything works, it's a great feeling. Right?
Florian:Yeah. Beautiful. Cause they are beautiful things.
Matt:Yeah. Serendipity. Um, let's talk about attractors for a little bit. Right? Um, attractors are tricky to describe. So not coming from a mathematics background, it was very hard for me to understand what an attractor even is or what a dynamical system even is. Uh, I don't know if you can help explain that or not cause it's a hard thing to explain. You can try.
Florian:I think I can. Um, I think, I mean put like, you know, highly simplified, right. An attractor is quite simply if you have a, um, a system with a lot of elements, um, there's obviously the, the space in which that system can be in terms of all the Combinatorics, all the different elements can be in a, you know, a combined, span a wide space of what the configuration at any-
Matt:Did you call it a high dimensional space?
Florian:Yeah. So it's like a high dimensional space. You have like, I dunno, let's say like a hundred units and they can be on or off. That's a very big space in terms of what could be represented. Um, but the interesting thing is when you build, put some structure into this, uh, when you connect these nodes and you associate some things, then you can have substructures within that and more than that is that the, when you have sort of some number of connected notes, call them, for simplicity sake, um, then when you activate just some of them, they might recruit the rest of that, what we call an ensemble. So sometimes these terms, I use interchangably like, um, you know, like, like an attractor or an ensemble or, um, there's a couple of more times that escape me now. But the point is quite simply, sort of the, the first main important, um, characteristic of, of any attractors that they can do things like pattern completion. So whenever you get close to the state that is encoded in an attractor and it's that particular configuration of activation, then the network activity will be attracted to that configuration. Meaning it's gonna change to the interactions of the elements into a configuration that is that attractor state. Right? The cool thing about that is that you can have many of those in a big network. You can embed tens, hundreds, thousands of attractors and they rely on connections between your nodes, right these nodes inthe brain would be neurons, right? So you would get some kind of a population code where different configurations of active neurons together represent something. And then if you activate just a tiny piece or you get close in the activity space to one of the encoded attractors, then the network would fall into those attractors. Right? So you would, and then as a matter of research off to us, that is, well, I'll give you a cue and you retrieve the memory, right? That's why attractors are useful memory models because they are in fact-
Matt:You feel something fuzzy perhaps. And you match to fuzzy objects you've touched or something like that.
Florian:Something like that. Yeah. So, um, that already gets a little bit complicated because of course when there's many fuzzy objects, that might mean at any given point you might fall into different attractors and then becomes relevant. Well, what biasing elements do you have? So maybe you also, you know, see if certain color or certain shape or something. Context matters then, and then the bias is the evolution of the network when all these units activate and inactivate each other through their interactions into a certain direction. Right? Um, the little weird thing about sort of like these dynamical attractors is that they don't just fall into a place like the simplest forms of attractor networks, like so-called Hockfield networks, right? They have a number of embedded attractors and as soon as they enter that attractor, the network is stable there so it will not change over time, right. In neural networks and in the kinds of, um, biophysically detailed spiking networks that I have built, um, attractors dynamically destabilize. That does not mean that theattractor is deleted from the memory. It's still in the network in the lasting connections. But neurons will, will tire out, they will wear out, which is just thing called neural adaptation. Uh, and the, the signaling chemicals that are available for immediate transmission will, will deplete. That is called synaptic depression. And so you can use those two elements to build a system that can fall into an attractor activated for awhile and then automatically be pushed out of it because the resources that are necessary for maintaining that item and activity get depleted, which then means the network is free again to wander around and find some other attractor.
Matt:Does that relate to short term memory at all? Like holding things in your mind and if you don't think about them while they just go away?
Florian:Uh, yes. No. So, uh, so the idea is there's only one thing on your mind at any given moment in time. You can jump between different things, but there's always sort of a foreground of activity, which we obviously relate to from the conscious experience of always attending to something that can be external, right? But it can also be internal when you're thinking of your own memories and what you might want to think of. And so it turns out that even though the brain is like this massively parallel system with all these neurons that are active at any given time, you're still playing it like a, like a keyboard. So there's only one, you know, configuration active at any given point in time, even though it's capable of many different ones. And some of them are built to transition. Like when you're learning sequences, one series of three tones activates the next activates the next etc. So sequence memory, right. The thing that the HTM neuron model at Numenta really excels at, um, these, these kinds of memories are also a form of attractor because the activity is pulled forward into something. It's just that that attractor is not one fixed point cause that point immediately it evolves into something else.
Matt:It's initiated by well it doesn't, not always, but potentially initiated by sensory input to roll through one of these attractor sequences. And then you're constantly comparing sensory input to the sequence if you're playing a song or just singing along with something or whatever.
Florian:Yeah. The system does that by itself.
Matt:So like there's constantly attractors going on in your head from one thing to the next. And this could represent any number of things, right?
Florian:Like essentially called the cortical attractor theory of neocortex or neocortical memory. The idea being that you have these distributed representations or specific brain states that actually get encoded, right? So there's strong connections between them, right? And they are competing for activation at any given moment in time. Some of them are associated with one another so they can activate each other go forward or backward. Um, and one of the nice ways to embed these memories into a system like that is to use Hebbian learning roles, right? Or in my case it's specific Bayesian um, uh, derived Bayesian statistics derived Hebbian learning rules. So which is in some sense optimal because you're not just computing correlation between two units, but you're also normalizing that by the, by the prior stuff, the pre and the postsynaptic neuron, which is a bit of technical detail I guess. But for those interested in Bayesian models, that might be interesting.
Matt:Um, okay. Let's talk about the binding problem, uh, specifically when I was reading your third paper in your thesis about the short term memory versus longterm memory areas. Um, what, what is this binding problem? It's a big thing in neuroscience. And, and how, how do, how do you think it should be addressed?
Florian:Right? Um, so there's a lot of in the, the, one of the problems of, uh, of my work right? And the, the, the field that I work in, it's, um, that it's very, um, it's very interdisciplinary, right? So there's ideas from cognitive science where the binding problem comes from, which I get to a second. There is obviously a neuroscientific evidence on all kinds of things and details that constrain models when you build them, and then there's the whole computational side. How do you build these systems and neural network simulators and how do you put these things together? And as a consequence, my work tries to speak to all of these different groups, right? I want to build systems that are reasonably defensible but thsy also do something useful computationally. Um, and that also, you know, sort of demonstrate what we can do in terms of a brain simulation, but also do something cognitively interesting.
Matt:Have some utility.
Florian:All right. And so the, the, the, one of the kinds of, uh, of the binding problems is this what's called role filling. So I think I use this example in, in that paper of I'm telling you that the, the, the, the name of my parrot, maybe Charlie. And so, you know, Charlie normally has a name of somebody, right? You might have a friend called Charlie, it's just a label, right? But if I now tell you when, after telling you that the name of my parrot is Charlie, you won't be like, you won't be surprised if I tell you that, you know, Charlie can fly and you might even be able to tell me now that Charlie can probably speak because you've now connected these elements. And the interesting thing is you don't have a longterm memory representation of that because you literally just learned that and the kinds of longterm memory that we're talking about with the LTP- that takes at the very least hours to express. Um, so you cannot have a brain structure that represents a flying parrot called Charlie, but you're still capable of making that association very quickly.
Matt:Yeah. Right away. I can think about it.
Florian:Exactly. So like you, you know, you do these memory talks about you, people just, you flash them out a card for like a fraction of a second, then they can tell what card did you just see? Well, it'ss the ACE of spades. Okay, cool. Um, so turns out that associative binding can be very quick and then you really have to ask where does that binding take place? And one of the ways that people used to think about that in terms of persistent activity is that all of these things get activated together. So the name Charlie, right, with or without your friend that has that name.
Matt:That could be perhaps in a language area.
Florian:Exactly. Be all active together. Yeah. The problem was that is that, um, that is nice if it's one memory or two, but if you go now doing a working memory task and you're holding on to other things like we are talking about all kinds of concepts here and you still know that the name of my parrot is Charlie, right? Um, and so, um, somehow we must be able to buffer these things even when the activity for them becomes undetectable. There's all these wonderful studies about activity, silent working memory, uh, that have come out in recent years, um, of people who've been looking for the signatures and finding them. So you can read out the content of the working memory, but there's the substantial period when you distract people. Or when people have to shift their attention to a multiple items they have to hold on to where the entire activity signature of that working memory item gets lost. You cannot get detected as soon as you provide a clue. It's back again.
Matt:Yeah, I know what you mean. As a software engineer, I know what it's like to be deep in a coding project and get interrupted and come back and have no idea what I was doing. But then as soon as I find that nugget, I'm like, Oh, that's okay and I'll get right back where I was. It's like your short term memory is pulling everything from longterm memory and then putting it together.
Florian:and so the, so the answer clearly must be, at least in my understanding of it, that if the, if the encoding is not in the activity space, even though it's often visible in the recordable activity of neurons, then if it's going to be silent for some while the information must go somewhere. Yeah.
Speaker 2:And the only place in the brain where information can go that is not sort of active, you know, in the neural activity. Well it's in the changes in the, in between the neurons, the synapses. Yeah. So that must be very fast, right? Because again, I've flushed your card and put it down and you already know what it was. Yeah. It must be very fast buffers. That might be activity dependent and reasonably fast non-activity dependence, synaptic changes, and if they are going to be associative, they're likely Hebbian in some form. Yeah, and the cool thing about those very fast forms of Hebbian plasticity as a mechanism to solve this binding problem to put things together, right, is that you don't have to record the full context. You already know what a parrot is. You're all familiar with that. Charlie is a name, it's not some weird phonetic sequence. Right?
Matt:But you need a long connection, right. For somehow from the short term memory area.
Florian:Yeah. The problem was that the, these representations might be far apart. Right? They might not just be neighboring assemblies that now can immediately click together.
Matt:You've got a sound of a parrot in the auditory area versus the visions of parrots.
Florian:Exactly. You somehow need to put them together and the brain can build, you know, like these powerful associations across neocortex. But again, if it's longterm structures, like your longterm memory, it's going to take time. And if we buy the story of longterm memory conservation during deep sleep in hippocampus, that may takes hundreds of reactivations during deep sleep. So you need some other mechanisms while you're awake and behaving to do, to solve this binding problem. And so the solution that we've come up with is this idea that prefrontal cortex does not actually hold the full content of working memory. All that it needs to do is to hold a temporary short term index with which those things can be linked. And I've shown in my work that the connectivity that is required for that can actually be very low. You don't need a lot of silences, um, particularly when the items that you want to bind together are very strongly encoded. So you already know that geometric shape and you already know that, you know, phonetic that that word or that sound right. So these are very strong assemblies that will self complete if you give them a tiny piece of it. So you don't need much information, but you need to direct the bias that makes sure that when you now see the shape or full name or whatever, you know, things you are associating, you are capable of bridging, right. Only a few brain areas that are situated in a way that they could do that.
Matt:So let me restate this and make sure I understand it properly. In the short term memory area of the prefrontal cortex, all you really need to create an associative, associative links between objects when you're putting together working memory is just enough to kickstart the attractors, right? And so just a, just a nugget of it so that they attractor can be invoked in whatever other parts of the brain and then an association between those nuggets, right?
Florian:So they become active together.
Matt:And then if, if in longterm memory, if you have a sensory input that comes through sensory cortex, that can trigger your short term memory to invoke the whole association. Yeah.
Florian:Right. So I do this example right where I a have a very brief cue which activates one longterm memory representation through sort of sensory input. Then that thing is how it activates an index in prefrontal cortex and retrieves an associated longterm memory in a brain area that might not, you know, might be far away like we're talking centimeters away here, right? So where there's very unlikely to be direct connections between the things that you're wanting to associate, but we know that the working memory is reasonably universal. You can- of all the things, you can have in longterm memory, you can associate almost anything. Yeah. Um, so since there can't be a dedicated population for any conjunction of things you might be able to come up with because the permutative complexity is too high, um, you need a flexible system. And so a fast, Hebbian mechanism might do just that. Luckily enough, last, there's some early findings of such mechanisms. Again, they're hard to observe because they're so fleeting. Particularly many of the old experimental paradigms that like tried to measure synaptic plasticity all the time by pinging the synapse. They might actually delete that encoded stage very quickly so that you might be blind to the, to the observation you want to make because you could essentially by looking, you are deleting it. It's a little bit like the, like the example of shooting a cat, right? Right. Um, like the cat is alive and a dead dad at the same time, but you can't look, uh, as soon as you look at it's either one or the other. This is a little bit like that because if you want to observe it, if you test the synapses and not by a lot of pings to see what its strength is, then you're eroding whatever, you know, it's coded state was. So silence is like, you know, the way that working memory might get preserved, not activity.
Matt:Interesting. Well, I think, uh, if anybody wants to know more about any of this stuff, uh, your, your thesis is a great place to start. Once again, active memory processing on multiple timescales and simulated cortical networks with Hebbian plasticity. Maybe there's an acronym we can make out of it.
Florian:Yeah. People are fighting over the nomenclature for this so what is STP short term plasticity, you know, some people are now arguing, well, you know, it might be STSP, um, because you want to differentiate, you know, different subclasses of that. And then some people call it[inaudible] longterm potentiation because it's lasting, but it's fragile. So there's a zoo of new terms, um, and it's a little bit like the nomenclature on inhibitory neurons. It's like a zoo and there's lots of different classification schemes and are just waiting for neuroscientists to agree on something, but we're not letting that stop us. We're building models anyways.
Matt:Oh, you're operating on the, essentially the frontiers of science here, so you're going to have that problem for sure.
Florian:Right. But that's also makes it exciting, right? I get to read a lot of what is happening and experimental notice science. I'm always of course hunting for what is the consensus, what is, what are the mechanisms that might be useful? What are the missing pieces on that that makes it very exciting day to day.
Matt:Yeah, absolutely. Uh, well Florian, it was a pleasure. Uh, I should remind Watchers or, uh, listeners that if you want to hear more from Florian, you can go to our YouTube channel, the Numenta YouTube channel, where we have live research meetings. Florian's given a couple of those recently, as you mentioned earlier. And uh, there'll probably be more, I'm sure there will be more. Yeah. Yeah. So I can get more Florian there. Um, thanks again. Fist bump, it's what we're doing, all right. Thanks for watching everybody. Thank you. Thanks again for listening to the Numenta On Intelligence podcast. I am Matt Taylor from Numenta. You can get more at our YouTube channel. Just search YouTube for N umenta and also follow us on Twitter at Numenta N. U. M. E N. T. A.